Executive Insights: Data Quality Is an Interesting Topic, I Swear!
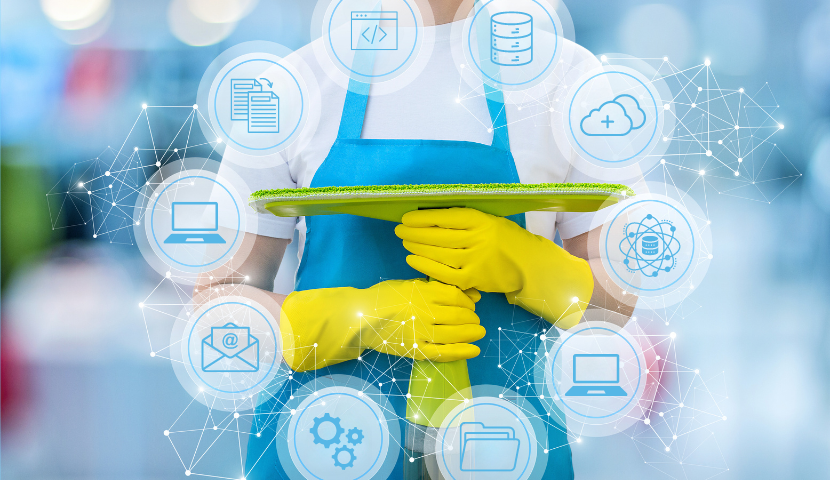
Steve Jacobson
Chief Executive Officer
I must admit that, when I was approached by our marketing team to write a blog post about data quality, I was pretty skeptical.
After all, how can anyone take a subject like data quality and make it interesting enough to read through 800 or so words. Well, challenge accepted and I’m about to give it a shot.
A Neglected Metric
First off, do we know what data quality actually is?
We know what water quality is, but how do we define data quality? When the Environmental Protection Agency (EPA) looks at water quality, they have set standards for what makes water safe for drinking.
We data nerds, on the other hand, have no such standards for what makes data safe for our consumption.
So, is data quality a vague concept, or can we actually break down data quality into specific properties or factors that make it “bad” or “good”?”
Data Quality Watchlist
Duplicate Data
Let’s start with duplicate data. That sounds pretty bad if you have it. But, given all of the different sources of data that come funneling into a nonprofit’s CRM, having zero duplicate records seems like the holy grail.
To get there, though, our first step might be to run a duplicate checking report where you would compare data between two records to see if they’re the same.
First on that list would be looking at the email addresses, to see if they’re the same. If you find two records with the same email address, you likely have a duplicate.
Keys
However, as we don’t always have everyone’s email address, we’ll need to dig deeper. A good way to do this is to build a key that consists of various bits of data.
For example, we could take the first three characters of the first name, the first four characters of the last name, the number of the street address and the first five characters of the city. We glue them together to create our key and we then compare that key across records to identify more duplicates.
You can keep on building keys and checking data until you feel that you have a good handle on them. You could then go into your CRM and merge the duplicate records.
Coded Fields
Another aspect of data quality relates to how the data is coded in your CRM.
At JCA, we have built a Codes Frequency Report (CFR) that, for coded fields, lists the code and its respective description along with a count of how many times that specific code has been used in the system.
We will also include the last time the code was used in the system if the CRM tracks that. The CFR allows you to identify codes that mean the same thing and therefore could be combined. It also identifies codes that have only rarely been used in the system and therefore could either be deleted or remapped to another code that means the same thing.
And, for codes that haven’t been added or used in years, they might be obsolete and are ripe for deletion.
Processes
In addition to coded fields, you’ll want to look at processes that create those codes. You may find that, for instance, marking someone as deceased was coded one way 10 years ago, another way five years ago and is different today.
We liken this phenomenon to an archaeological dig where you have different layers of data “sediment” that should be fixed so there’s consistency throughout the database.
Captured Data
While we tend to think of data quality in the negative (my data is so bad!), we also need to keep in mind that data quality is also dependent on capturing—and effectively managing—data that is not only clean, but useful and actionable.
For example, if you want to understand the age distribution of those people attending your events, you need to store their birthdates.
But, if you don’t have a use for keeping birthdates in your system, don’t expend the effort. Make sure that whatever you’re tracking is useful and actionable.
Leveling Up
So, now that you’ve cleaned up all your data, what can you expect?
First off, your reports will be more accurate, consistent and (hopefully) actionable. This will allow you to gain new insights from your data and more effectively measure your team’s performance.
Going forward, you may want to create a data governance team to establish and enforce data management policies.
Much like we want to leave clean water for the next generations, we also want to leave clean data for your future organization.
Data Cleansing
Know ahead of time exactly what it will cost to cleanse your data and make sure your most critical cleansing is completed first.
We produce a detailed list of the cleansing issues identified by our Codes Frequency Report during discovery (coding issues, and report and output needs), then create a detailed plan to resolve even your most complex issues.
Don’t let poor data quality hold you back. Contact us to learn how we can help.